Demand Prediction in Pharma Supply Chains Using Time Series Analysis and Forecasting Tool
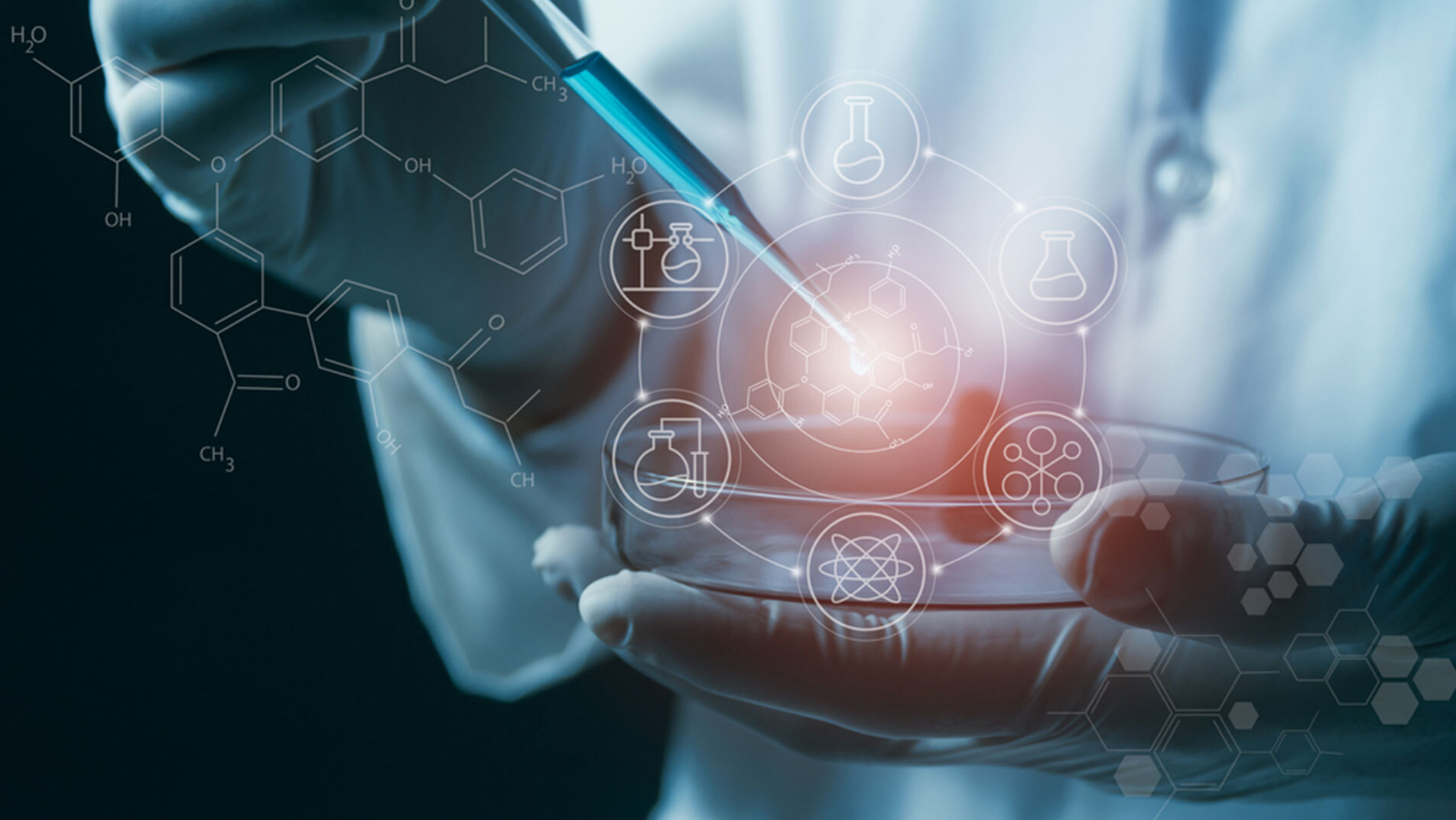
According to the US Federal Government, pharmaceutical companies invest 25% of their revenue in medical research and discovery. In addition, the overall pharmaceutical industry is projected to reach $1,163 a billion in 2023 due to an exponential increase in pharma demands.
Demand confirms the required quantity of a product to consumers and demand prediction helps stakeholders to keep supply and demand in balance. Therefore, time series analysis and forecasting techniques are used to predict risks in pharma but let’s first understand what the term “time series analysis and forecasting” means.
Time Series Analysis and Forecasting
Time series analysis is used in a variety of fields such as Pharmaceutical, Telecommunication, Power, and Energy to forecast future trends, identify patterns and relationships, and detect anomalies in the data. This type of analysis is particularly useful in situations where past data can provide insight into future performance.
Several techniques can be used in time series analysis and forecasting. One popular method is exponential smoothing, which uses a weighted average of past observations to make predictions. Another technique and machine learning model is ARIMA (Autoregressive Integrated Moving Average), which learns the underlying structure of the time series data and makes predictions based on the learned pattern.
Time series analysis and forecasting is a challenging task, as it requires a deep knowledge of statistical methods and the ability to interpret patterns in the data. However, the insights gained from this type of analysis can be invaluable for businesses and organizations looking to make informed decisions about the future. However, by comprehending the patterns and trends in their data, organizations can make more accurate predictions, optimize operations, and stay ahead of the competition.

How can Pharma Supply Chain be Improved?
One way time series analysis improves the pharma supply chain is by predicting demand for drugs. By analyzing historical data, organizations can identify patterns and trends in drug sales and use this information to forecast future demand. It also ensures that the right amount of drugs is produced, minimizing the risk of shortages or overproduction.
Another way time series analysis can leverage the pharma supply chain is by predicting lead times (Medicines Planning, Production, and Inventory) for drug production and delivery. By analyzing data on the time it takes to manufacture and deliver drugs, organizations can understand patterns and trends in lead times. This information is used to improve supply chain planning in organizations on a massive scale.
In addition, time series analysis can also be used to detect anomalies in the pharma supply chain. By analyzing data on drug shipments and inventory levels, organizations can identify unusual patterns or events that may indicate issues such as theft, counterfeiting, imbalance demands or other supply chain disruptions.

Meet Demands by Forecasting Pharma Data
Time series analysis involves analyzing historical data on drug sales and identifying patterns and trends. These patterns are used to forecast future demand for drugs, allowing pharmaceutical companies to plan their production and distribution accordingly.
In addition to it. There are other factors that can be included in the time series forecasting models. Such as, changes in the population, economic factors, and disease prevalence rates. By incorporating these additional factors, pharmaceutical companies can create more accurate demand forecasts using machine learning techniques. This can result in better patient outcomes and a more effective pharma demand management.
Better Demand Prediction with Odyx yHat
Odyx yHat is a powerful time series forecasting tool for demand prediction in the pharma industry. It is a machine-learning platform that uses advanced algorithms to analyze historical data and generate accurate forecasts on future demand.
One of the key advantages of Odyx yHat is its ability to incorporate multiple data sources into its demand prediction models. By analyzing this data in real-time, Odyx yHat can generate more accurate demand predictions and provide pharma companies with the information they need to optimize their production as well as distribution processes.
Moreover, Odyx yHat uses modern machine-learning techniques to forecast on real-time data from a data warehouse or a data lake. This means that as new data becomes available, this time series tool will continue to refine its demand prediction models and provide even more accurate forecasts.

Takeaway
Frequently Asked Questions
How does time series analysis help stakeholders?
Time series analysis helps stakeholders in the pharma industry to predict demand, optimize inventory, monitor drug quality, ensure regulatory compliance, and make data-driven decisions. It improves operations and patient outcomes.
Why should you use time series analysis and forecasting tool?
Using time series analysis and forecasting tools like Odyx yHat in the pharmaceutical sector provides accurate demand forecasting, better inventory management, improved production planning, and enhanced supply chain management. It helps stakeholders make data-driven decisions and optimize operations to improve patient outcomes.
Need Our Help?
Odyx yHat reciprocates your core analysis needs for making an insightful decision with respect to business needs. Time series forecasting tool to solve challenging industrial problems while turning the complex process hassle-free.